検査の知識
MRI検査とMRA検査の違い
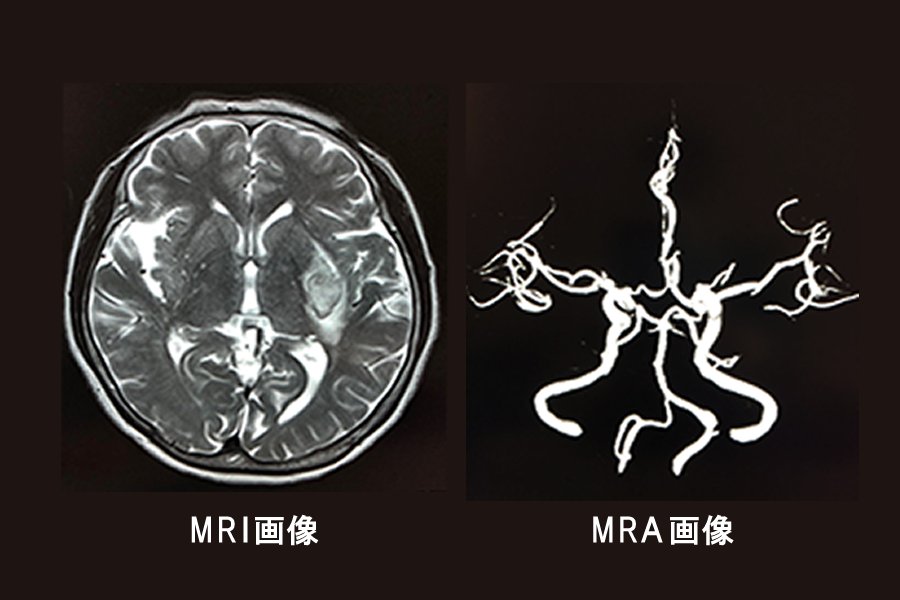
脳ドックは放射線被曝のない安全な検査です。
- MRI検査では『脳全体』を視覚化して、『脳卒中(脳梗塞・脳出血・クモ膜下出血)の潜在』や『認知症の進行を示す脳の委縮』などの早期診断が可能です。
- MRA検査では『脳の血管の細部まで』を視覚化して、『脳梗塞の原因になる動脈の狭窄』や『クモ膜下出血の原因となる未破裂脳動脈瘤』などの早期診断が可能です。
頸動脈(首)超音波で何が分かるの?
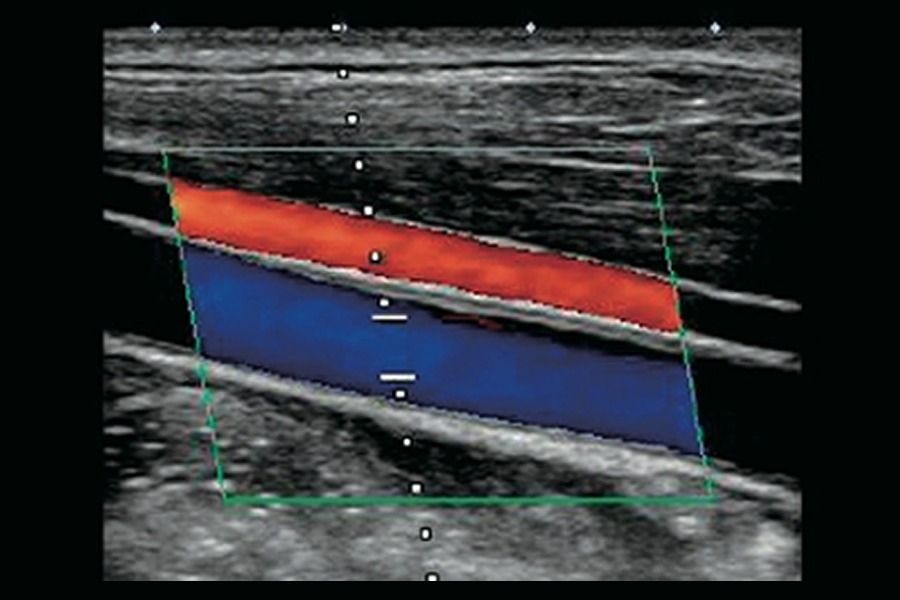
首の血管状態=全身の血管状態であるため、頸動脈(首)の超音波を行っています。
脳の専門クリニックにおいて、血管の状態、つまり動脈硬化の進行具合を確認することは大変重要です。動脈硬化が進行すると、血管壁が硬くなり弾力性が失われ、壁の内側にコレステロールなどがたまり、脳の血管が詰まる『脳梗塞』などの原因となるためです。
MRI画像で 早期の認知症変異を抽出!
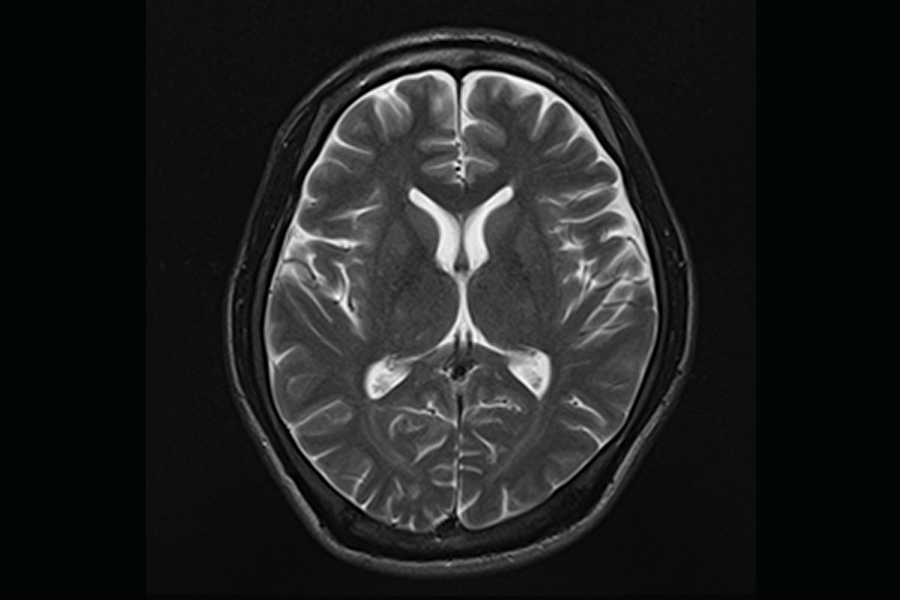
脳画像は認知症の早期変異を抽出するために有益かつ不可欠な検査です。
野々村クリニックは、日本脳ドック学会認定施設外部サイトでもあり、多くの症例と照らし合わせたわずかな変異を見逃さない診断をモットーとしています。
脳ドックにAI技術『AiCE』導入
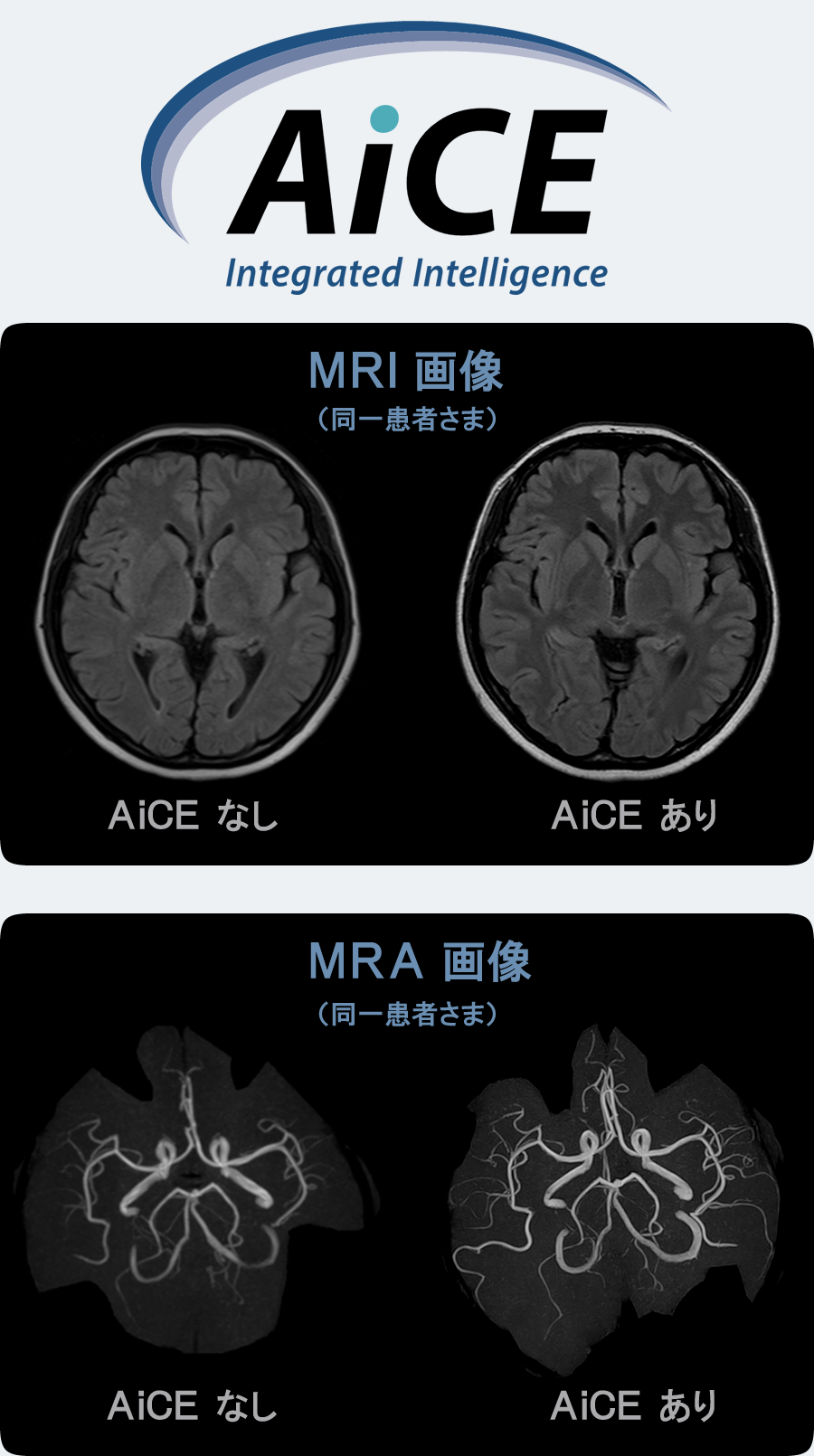
野々村クリニックでは、信頼して来院してくださる患者さんのために、AI技術を積極的に導入しています。
キャノンが開発したAiCE(Advanced intelligent Clear-IQ Engine)は、Deep Learning(※1)を用いて膨大なMRI画像を人工知能が常に学習し、ノイズ(不要な部分)を除去する技術です。
AiCEはノイズ成分と信号成分を識別する処理を用い、空間分解能を維持したままノイズを選択的に除去することが可能で、MRI機器がもっている時間分解能を最大限に引き出しながら、高いノイズ低減効果を得ることができます。
低コントラスト領域においても、粒状性を維持しながら高いノイズ低減効果が得られ、低線量領域でも安定した画質向上を実現しています。
(※1)Deep Learning(深層学習)はAIを実現するためのアプローチの一つです。
AIは機械が再現する人工知能(Artificial Intelligence)です。それを実現するためのアプローチがMachine Learning(機械学習)であり、このうちの一つにDeep Learning(深層学習)があります。Deep Learning(深層学習)は昨今急速に進化を続けるAIにおいて基盤となる重要な技術です。
この技術により、検査時間を短縮できるだけでなく、飛躍的に画像が良くなりました。
患者さんに優しく、医師にとってもさらに正確な診断が可能になる技術の導入を積極的に行っています。
より詳しい情報は、キャノンメディカルシステムズ株式会社外部サイトのサイトをご覧ください。
- 1)Isogawa.K,Ida.T,Shiodera.T,Takeguchi.T
Deep Shrinkage Convolutional Neural Network for Adaptive Noise Reduction
IEEE Signal Processing Letters, Vol.25, No.2, February 2018. doi.:10.1109/LSP.2017.2782270 - 2)Kidoh.M,Shinoda.K,Kitajima.M,Isogawa.K,Nambu.M,Uetani.H,Morita.K,Nakaura.T,Tateishi.M,Yamashita.Y,Yamashita.Y
Deep Learning Based Noise Reduction for Brain MR Imaging: Tests on Phantoms and Healthy Volunteers
Magnetic Resonance in Medical Sciences. September 4, 2019.doi:10.2463/mrms.mp.2019-0018 - 3)Moreno RA, De Sá Rebelo MFS, Carvalho T, Assunção AN, Dantas RN, do Val R, et al.
A combined deep-learning approach to fully automatic left ventricle segmentation in cardiac magnetic resonance imaging SPIE Medical Imaging. 2019. doi.org/10.1117/12.2512895 - 4)Mori R, Ota H, Masuda A, Kimura T, Nagasaka T, Nishina T, et al.
Ultrashort TE Time-Spatial Labeling Inversion Pulse MR Angiography Denoised with Deep Learning Reconstruction for Abdominal Visceral Arteries : A Feasibility Study.
International Society for Magnetic Resonance in Medicine. 2019. First published: 26 December 2020 https://doi.org/10.1002/jmri.27481 - 5)Yokota Y, Takeda C, Kidoh M, Oda S, Aoki R, Ito K, et al.
Effects of Deep Learning Reconstruction Technique in High-Resolution Non-contrast Magnetic Resonance Coronary Angiography at a 3-Tesla Machine.
Can Assoc Radiol J. 72(1):120–7. doi: 10.1177/0846537119900469. Epub 2020 Feb 19. - 6)Mournet S, Okubo G, Koubiyr I, Zhang B, Kusahara H, Prevost VH, et al.
Higher b-values improve the correlation between diffusion MRI and the cortical microarchitecture. Neuroradiology.2020 Nov;62(11):1411-1419. doi: 10.1007/s00234-020-02462-4. Epub 2020 Jun 1. - 7)Sagawa H, Fushimi Y, Nakajima S, Fujimoto K, Miyake KK, Numamoto H, et al.
Deep Learning-based Noise Reduction for Fast Volume Diffusion Tensor Imaging: Assessing the Noise Reduction Effect and Reliability of Diffusion Metrics Magn Reson Med Sci . 2020;771:1–7. https://doi.org/10.2463/mrms.tn.2020-006 - 8)Taguchi S, Tambo M, Watanabe M, Machida H, Kariyasu T, Fukushima K, et al.
Prospective Validation of Vesical Imaging-Reporting and Data System (VI-RADS) Using a Next-Generation Magnetic Resonance Imaging Scanner: Is Denoising Deep Learning Reconstruction Useful?
J Urol. 2021 Mar;205(3):686-692. doi: 10.1097/JU.0000000000001373. Epub 2020 Oct 6. - 9)Graves C V., Moreno RA, Rebelo MS, Nomura CH, Gutierrez MA.
Improving the generalization of deep learning methods to segment the left ventricle in short axis MR images. 2020 42nd Annu Int Conf IEEE Eng Med Biol Soc. 2020;1203–1206. DOI: 10.1109/EMBC44109.2020.9175256 - 10)Kato.Y, Bharath.A, Kassai.Y, Larry.K, Joanne.S, Karan.K, Shelton.C, Joao A. C. Lima
Non-contrast coronary magnetic resonance angiography: current frontiers and future horizons
Magnetic Resonance Materials in Physics, Biology and Medicine volume 33, pages591–612 (2020). https://doi.org/10.1007/s10334-020-00834-8 - 11)Uetani H, Nakaura T, Kitajima M, Yamashita Y, Hamasaki T, Tateishi M, et al.
A preliminary study of deep learning-based reconstruction specialized for denoising in high-frequency domain: usefulness in high-resolution three-dimensional magnetic resonance cisternography of the cerebellopontine angle
Neuroradiology. 63, pages63–71 (2021). https://doi.org/10.1007/s00234-020-02513-w - 12)Ueda.T, Ohno.Y, Yamamoto.K,Iwase.A,Fukuba.T, Hanamatsu.S, Obama.Y, Ikeda.H, Ikedo.M, Yui.M, Murayama.K, Toyama.H
Compressed sensing and deep learning reconstruction for women's pelvic MRI denoising: Utility for improving image quality and examination time in routine clinical practice
European Journal of Radiology 134 (2021) 109430. doi:10.1016/j.ejrad.2020.109430 - 13)Tajima.T, Akai.H, Sugawara.H, Yasaka.K, Kunimatsu.A,Yoshioka.N, Akahane.M, Ohtomo.K,Abe.O,Kiryu.S
Breath-hold 3D magnetic resonance cholangiopancreatography at 1.5 T using a deep learning-based noise-reduction approach: Comparison with the conventional respiratory-triggered technique
European Journal of Radiology 144 (2021) 109994. doi.org/10.1016/j.ejrad.2021.109994 - 14)Naganawa.S, Ito.R, Kawai.H, Kawamura.M, Taoka.T, Sakai.M, Ichikawa.K, Yoshida.T, Sone.M
MR Imaging of Endolymphatic Hydrops in Five Minutes.
Magnetic Resonance in Medical Sciences.April 24, 2021. https://doi.org/10.2463/mrms.ici.2021-0022 - 15)Takeshima H.
Aliasing layers for processing parallel imaging and EPI ghost artifacts efficiently in convolutional neural networks. Magnetic Resonance In Medicine. Volume86, 820-834. 14 March 2021, https://doi.org/10.1002/mrm.28758 - 16)Ueda.T, Ohno.Y, Yamamoto.K, Murayama.K, Ikedo.M, Yui.M, Hanamatsu.S, Tanaka,Y, Obama.Y, Ikeda.H, Toyama.H Deep Learning Reconstruction of Diffusion-weighted MRI Improves Image Quality for Prostatic Imaging
Radiology. 2022 May;303(2):373-381. doi: 10.1148/radiol.204097. - 17)Watanabe.M , Taguchi.S, Machida.H, Tambo.M, Takeshita.Y, Kariyasu.T, Fukushima.K, Shimizu.Y, Okegawa.T, Fukuhara.H, Yokoyama.K Clinical validity of non-contrast-enhanced VI-RADS: prospective study using 3-T MRI with high-gradient magnetic field
European Radiology https://doi.org/10.1007/s00330-022-08813-4 - 18)Tajima.T, Akai.H, Sugawara.H, Furuta.T, Yasaka.K, Kunimatsu.A, Yoshioka.N, Akahane.M, Abe.O, Ohtomo.K, Kiryu.S
Feasibility of accelerated whole-body diffusion-weighted imaging using a deep learning-based noise-reduction technique in patients with prostate cancer
Magn Reson Imaging. 2022 Oct;92:169-179. DOI: 10.1016/j.mri.2022.06.014 - 19)Matsuyama.T, Ohno.Y, Yamamoto.K, Ikedo,M, Yui.M, Furuta.M, Fujisawa.R, Hanamatsu.S, Nagata.N, Ueda.T, Ikeda.H, Takeda.S, Iwase.A, Fukuba.T, Akamatsu.H, Hanaoka.R, Kato.R, Murayama.K, Toyama.H
Comparison of Utility of Deep Learning Reconstruction on 3D MRCPs Obtained with Three Different k-Space Data Acquisitions in Patients with IPMN Eur Radiol. 2022 Jun 10. doi: 10.1007/s00330-022-08877-2. - 20) Akai.H, Yasaka.K, Sugawara,H, Tajima.T, Akahane.M, Yoshioka.N, Ohtomo.K, Abe.O, Kiryu.S
Commercially available deep learning reconstruction of 1.5T knee MRI has higher image quality than 3T MRI: a normal volunteer study.
Magn Reson Med Sci. 2022 Jul 9. doi: 10.2463/mrms.mp.2022-0020. - 21)Obama.Y, Ohno.Y, Yamamoto.K, Ikedo.M, Yui.M, Hanamatsu.S, Ueda.T, Ikeda.H, Murayama.K, Toyama.H
MR imaging for shoulder diseases: Effect of compressed sensing and deep learning reconstruction on examination time and imaging quality compared with that of parallel imaging
Magn Reson Imaging. 2022 Aug 4;S0730-725X(22)00136-9. doi: 10.1016/j.mri.2022.08.004. - 22)Yasaka.K, Tanishima.T, Yuta Ohtake.Y, Tajima.T, Akai.H, Ohtomo.K, Abe.O, Kiryu.S
Deep learning reconstruction for 1.5T cervical spine MRI: Effect on interobserver agreement in the evaluation of degenerative changes
Eur Radiol. 2022 Sep;32(9):6118-6125. doi: 10.1007/s00330-022-08729-z. Epub 2022 Mar 29. - 23)Kakigi.T, Sakamoto.R, Tagawa.H, Kuriyama.S, Goto.Y, Nambu.M, Sagawa.H, Numamoto.H, Kawai Miyake.K, Saga.T, Matsuda.S, Nakamoto.Y Diagnostic advantage of thin slice 2D MRI and multiplanar reconstruction of the knee joint using deep learning based denoising approach
Sci Rep. 2022 Jun 20;12(1):10362. doi: 10.1038/s41598-022-14190-1. - 24)Tajima.T, Akai.H, Sugawara.H, Furuta.T, Yasaka.K, Kunimatsu.A, Yoshioka.N, Akahane.M, Abe.O, Ohtomo.K, Kiryu.S
Feasibility of accelerated whole-body diffusion-weighted imaging using a deep learning-based noise-reduction technique in patients with prostate cancer Magn Reson Imaging. 2022 Oct;92:169-179. doi: 10.1016/j.mri.2022.06.014. Epub 2022 Jun 27. - 25)Yasaka.K, Tanishima.T, Ohtake.Y, Tajima.T, Akai.H, Ohtomo.K, Abe.O, Kiryu.S
Deep learning reconstruction for the evaluation of neuroforaminal stenosis using 1.5T cervical spine MRI: Comparison with 3T MRI without deep learning reconstruction
Neuroradiology. 2022 Aug 3. doi: 10.1007/s00234-022-03024-6. - 26) Kariyasu.T, Machida.H, Takahashi.S, Fukushima.K, Yoshioka.T, Yokoyama.K
Denoising using deep-learning-based reconstruction for whole-heart coronary MRA with sub-millimeter isotropic resolution at 3T: a volunteer study Diagn Interv Radiol. 2022 Aug 23. doi: 10.5152/dir.2022.21291. - 27)Fujiwara.M, Kashiwagi.N, Matsuo.C, Watanabe.H, Kassai,Y, Nakamoto.A,Tomiyama.N
Ultrafast lumbar spine MRI protocol using deep learning–based reconstruction: diagnostic equivalence to a conventional protocol
Skeletal Radiology volume 52, pages233–241 (2023) doi.org/10.1007/s00256-022-04192-5